Location: Innovation Hall, room E100 + MS Teams, with some exceptions (see below).
Remote viewing: A link is sent 24h before the talk to subscribers of the STATATUVM ListServ.
Fall
October 8th
3:00 PM ET
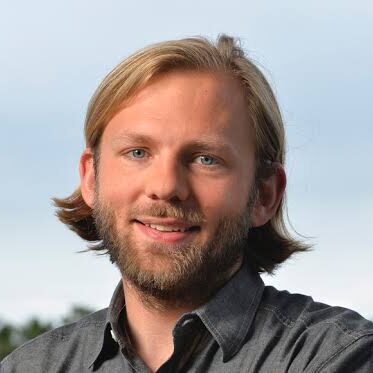
Johan Ugander
Stanford
Title: Harvesting randomness to understand computational social systems
Abstract: Modern social systems are increasingly infused with algorithmic components, designed to optimize various objectives under diverse constraints. Examples include content ranking algorithms in social media, review assignment algorithms in academic peer review, targeting strategies in social networks, and school choice mechanisms to assign students to schools. In many such systems (and in all of these examples), such algorithms are commonly randomized, motivated by fairness, strategic, or efficiency considerations. In this talk, I will argue for how such randomness can be harvested to make causal inferences not only about the effects of these systems on various outcomes, but also how the system would behave under alternative algorithmic designs, a version of off-policy evaluation. These evaluations come with unique methodological challenges, notably overlap that is often weak or nonexistent, but by developing novel methods to overcome these challenges we can gain confidence about the ways in which these systems operate and their impact on individuals and society as a whole.
November 7th
3:00 PM ET
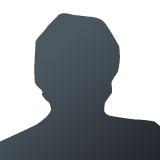
Pei Geng
University of New Hampshire
Title: Bias-corrected estimation in functional coefficient autoregressive models with measurement error
Abstract: The functional-coefficient autoregressive (FAR) models are flexible to fit nonlinear features in time series data with covariates. Cai et al. (2000) developed an effective local linear estimation procedure under the FAR framework. When the time series data are observed with measurement error, we first derive the asymptotic bias of the naïve local linear estimator (LLE) by ignoring the measurement error. Then, we propose a bias-corrected local linear estimation procedure for both the functional coefficients and the autoregressive error variance. Simulation studies shows superior performance of the proposed estimation method much reduced bias under various choices of FAR model settings. The asymptotic properties of the bias-corrected estimators are also established. At last, the proposed approach is applied to a cybersecurity real data example.
December 3rd
Location: Votey 209
3:00 PM ET
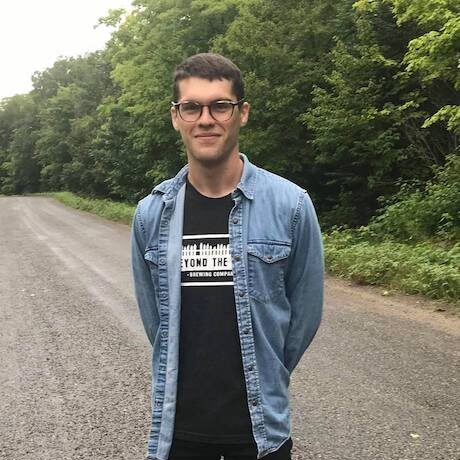
Peter W. MacDonald
University of Waterloo
Title: Mesoscale two-sample testing for networks
Abstract: Networks arise naturally in many scientific fields as a representation of pairwise connections. Statistical network analysis has most often considered a single large network, but it is common in a number of applications, for example, neuroimaging, to observe multiple networks on a shared node set. When these networks are grouped by case-control status or another categorical covariate, the classical statistical question of two-sample comparison arises. In this work, we address the problem of testing for statistically significant differences in a given arbitrary subset of connections. This general framework allows an analyst to focus on a single node, a specific region of interest, or compare whole networks. Our ability to conduct mesoscale testing on a meaningful group of edges is particularly relevant for applications such as neuroimaging and distinguishes our approach from prior work, which tends to focus either on a single node or the whole network. Our approach can leverage all available network information and learn informative projections that improve testing power when a low-dimensional latent network structure is present.
Spring
February 24th
Location: Innovation E210
3:00 PM ET
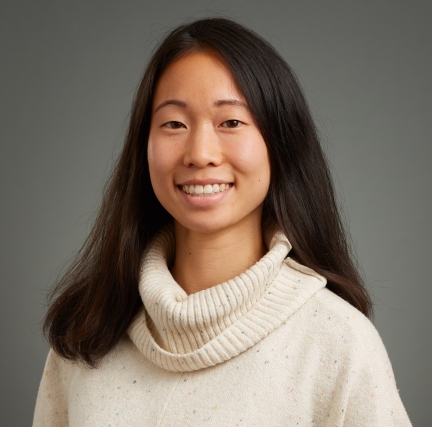
Becky Tang
Middlebury College
Title: Zero-inflated regression modeling for continuous data with applications in ecology
Abstract: A frequent challenge encountered in real-world applications is data having a high proportion of zeros. Focusing on ecological abundance data, much attention has been given to zero-inflated count data. Statistical models for non-negative continuous abundance data with an excess of zeros are rarely discussed. The work presented here considers the creation of a point mass at zero through a left-censoring approach or through a hurdle approach. Using a Bayesian approach, we incorporate both mechanisms to capture the analog of zero inflation for count data. Time permitting, we will also discuss zero-inflated modeling for multivariate abundance data. Applications may include percent cover of vegetation, tree biomass using FIA data, and insect abundance from urban streams in New England.
March 31st
3:00 PM ET
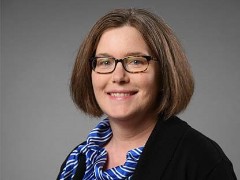
Erika Edwards
University of Vermont
Title: Investigating Outcomes for High-Risk Infants.
April 28th
Location: Innovation E210
3:00 PM ET
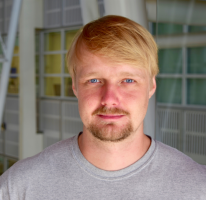
Jean-Gabriel Young
University of Vermont
Title: Designing interventions with message passing on clustered graphs
Abstract: Models of contagions can describe many network dynamics, such as cascading failures in economic systems, information diffusion, and pathogen transmission. These models are particularly helpful when designing intervention—for example, deciding how to optimally roll out vaccines or designing robust economic systems. The idea is, roughly, to use realistic contagion models to test counterfactuals, and optimize the system’s parameters to increase the likelihood of favorable outcomes. This is a difficult numerical optimization problem. A leading computational approach is message passing, which allows for the rapid and direct computation of the distribution over outcomes. In this talk, I will give a pedagogical introduction to message passing. I will then highlight some well-known issues with this framework, chiefly that it overestimates the likelihood that a contagion will spread. I will then discuss two promising approaches for correcting these issues: the neighborhood message passing (NMP) framework and graph machine learning techniques.
Past colloquia can be found here