Location: Innovation Hall, room E100 + MS Teams.
Remote viewing: A link is sent 24h before the talk to subscribers of the STATATUVM ListServ.
Fall
October 8th
3:00 PM ET
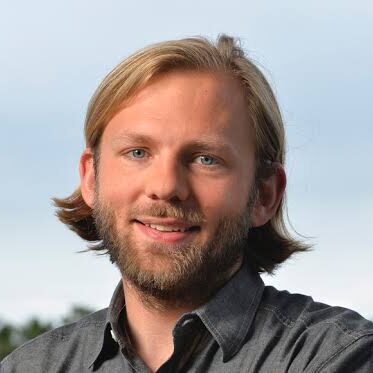
Johan Ugander
Stanford
Title: Harvesting randomness to understand computational social systems
Abstract: Modern social systems are increasingly infused with algorithmic components, designed to optimize various objectives under diverse constraints. Examples include content ranking algorithms in social media, review assignment algorithms in academic peer review, targeting strategies in social networks, and school choice mechanisms to assign students to schools. In many such systems (and in all of these examples), such algorithms are commonly randomized, motivated by fairness, strategic, or efficiency considerations. In this talk, I will argue for how such randomness can be harvested to make causal inferences not only about the effects of these systems on various outcomes, but also how the system would behave under alternative algorithmic designs, a version of off-policy evaluation. These evaluations come with unique methodological challenges, notably overlap that is often weak or nonexistent, but by developing novel methods to overcome these challenges we can gain confidence about the ways in which these systems operate and their impact on individuals and society as a whole.
November 7th
3:00 PM ET
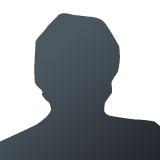
Pei Geng
University of New Hampshire
Title: Bias-corrected estimation in functional cofficient autoregressive models with measurement error
Abstract: The functional-coefficient autoregressive (FAR) models are flexible to fit nonlinear features in time series data with covariates. Cai et al. (2000) developed an effective local linear estimation procedure under the FAR framework. When the time series data are observed with measurement error, we first derive the asymptotic bias of the naïve local linear estimator (LLE) by ignoring the measurement error. Then, we propose a bias-corrected local linear estimation procedure for both the functional coefficients and the autoregressive error variance. Simulation study shows superior performance of the proposed estimation method much reduced bias under various choices of FAR model settings. The asymptotic properties of the bias-corrected estimators
are also established. At last, the proposed approach is applied to a cybersecurity real data example.
December 3rd
3:00 PM ET
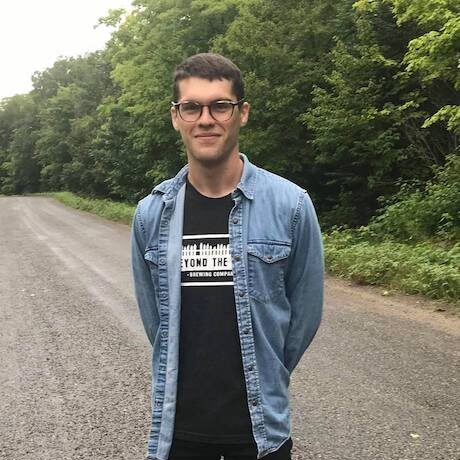
Peter W. MacDonald
University of Waterloo
Title: TBA
Abstract: TBA
Spring
TBA
Past colloquia can be found here