2023-2024 academic year
Fall
October 16th
3:30 PM ET

Sam Zhang
CU Boulder
Title: An illusion of predictability in scientific results: Even experts confuse inferential uncertainty and outcome variability
Abstract: Traditionally, scientists have placed more emphasis on communicating inferential uncertainty (i.e., the precision of statistical estimates) compared to outcome variability (i.e., the predictability of individual outcomes). Here, we show that this can lead to sizable misperceptions about the implications of scientific results. Specifically, we present three preregistered, randomized experiments where participants saw the same scientific findings visualized as showing only inferential uncertainty, only outcome variability, or both and answered questions about the size and importance of findings they were shown. Our results, composed of responses from medical professionals, professional data scientists, and tenure-track faculty, show that the prevalent form of visualizing only inferential uncertainty can lead to significant overestimates of treatment effects, even among highly trained experts. In contrast, we find that depicting both inferential uncertainty and outcome variability leads to more accurate perceptions of results while appearing to leave other subjective impressions of the results unchanged, on average.
November 6th
3:30 PM ET

Title: Feel the noise: a statistical journey through the resolution of a low frequency noise issue
Note: In nature, low-frequency noise (LFN) exposure is typically short-term and often serves as a warning (e.g., thunder). Modern society has sources of LFN that are pervasive, leading to prolonged exposure to this stressor. The WHO refers to LFN as an invisible toxin. I will discuss some of the challenges and successes of a project to remediate LFN. My interest in LFN research began six years ago due to some health issues. I was very fortunate to be able to join a team of acoustical and mechanical engineers to help design and implement a solution. The fact that everyone experiences sound differently, along with the clash between experimental design and practical considerations of operating a central heating plant, added to the complexity. In collaboration with the institution, I took the lead on writing the RFP that was used to hire two acoustical engineering firms to assess and remediate the source of the LFN. My talk will cover statistical aspects of the journey to a successful resolution of the LFN issue.
December 4th
3:30 PM ET

Leonard Stefanski
North Carolina State University
Title: Fractional Ridge Regression
Abstract: Ridge regression was introduced by Hoerl and Kennard (1970), and twenty-six years later was followed by the introduction of the lasso Tibshirani (1996). The body of research ensuing from these seminal papers is staggering and has contributed immensely to our understanding of shrinkage and selection methodology and to the practice of regression modeling in many areas of science. In some applications of regression modeling, the goal is simply to achieve the best possible predictions of future response values. In other applications, interpretation is important as a way to guide understanding of the process under investigation. Ridge regression is very good at prediction, although it is often eclipsed by the lasso in terms of both prediction and interpretation because the lasso also allows for selection.
The method introduced in this talk, fractional ridge regression, has the potential to improve both prediction (as measured by mean square error) and interpretability (as measured by the specificity of variable selection) relative to the lasso.
Spring
February 26th
3:45 PM ET
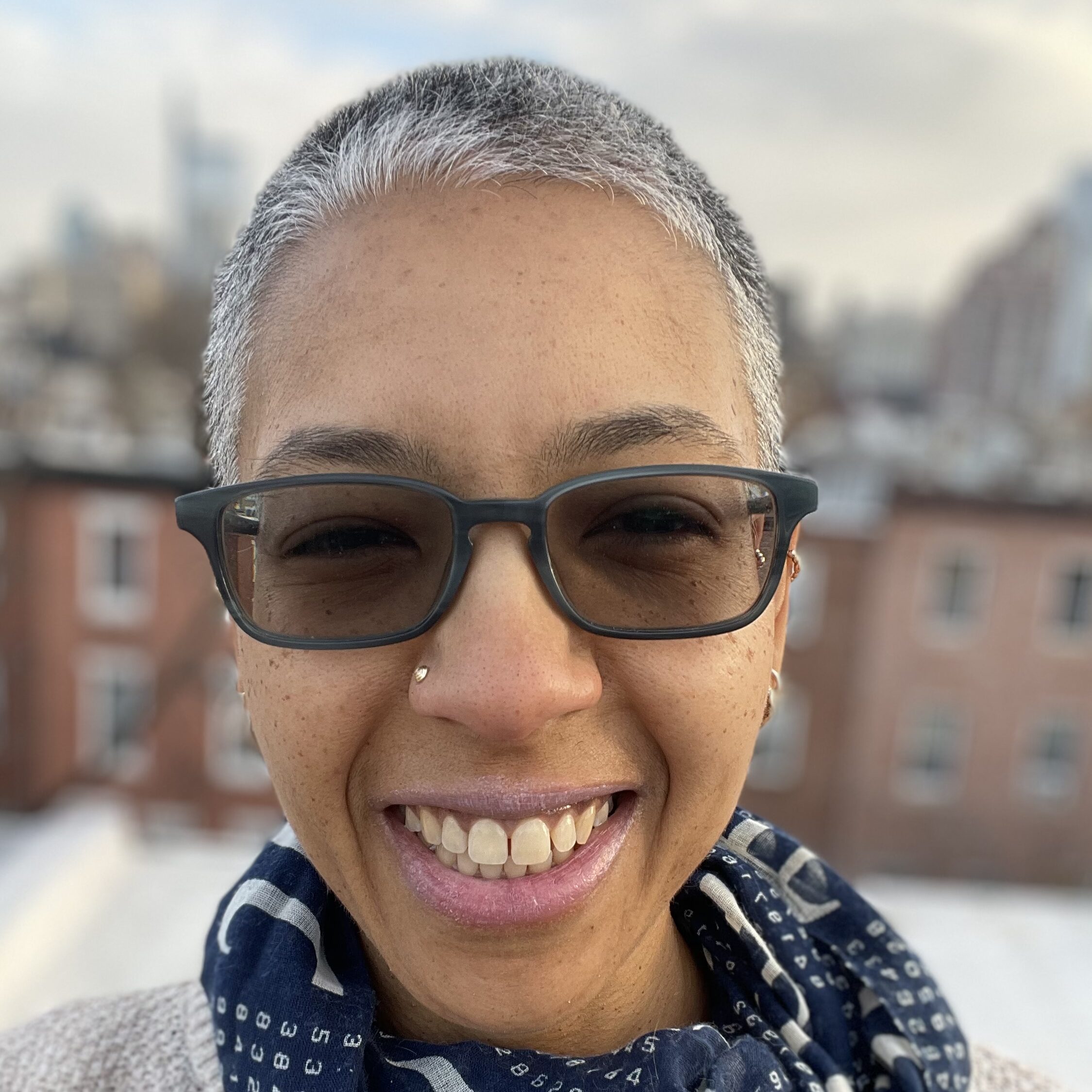
Rebecca Hubbard
University of Pennsylvania
Title: Opportunities and challenges for advancing health equity through electronic health records-based research
Abstract: Vulnerable populations, including racial and ethnic minorities and medically frail individuals, are under-represented in randomized clinical trials (RCTs), raising concerns about health equity and the external validity of results. Additionally, clinical trials may not reflect care and outcomes as they are experienced in routine practice and can be slow to provide timely evidence in the face of rapidly evolving or urgent public health or medical crises. Electronic health records (EHR) have the potential to address some of these concerns. However, limitations of EHR necessitate careful attention to study design and application of appropriate statistical methods. In this talk, I will discuss the potential of EHR data to supplement RCTs and support risk-guided medical decision-making by providing both more timely and generalizable evidence and presenting statistical approaches for addressing the challenges of conducting research in EHR data. Methodological approaches will be illustrated using real-world studies from the cancer care continuum. Through judicious application of appropriate methods, EHR have the potential to support more equitable health research, but careful consideration must be given to the limitations of this data source.
March 18th
3:45 PM ET
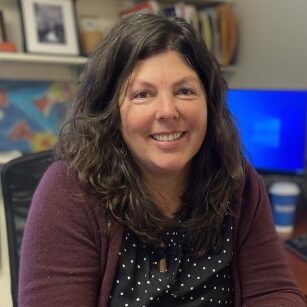
Abigail Crocker
UVM
Title: Addressing the harms of mass incarceration: Lessons learned from a 5-year community-engaged research effort.
Abstract: The US has the highest incarceration rate in the world and spends more than $89 billion on prison operations per year. Working or being confined in a prison has a negative impact on a person’s health. Both corrections officers and incarcerated individuals experience significantly higher rates of depression, post-traumatic stress disorder, and suicidal ideation compared to the national average. Moreover, the overall life expectancy for a corrections officer is reported to be 59 years, which is 16 years less than the national average. For incarcerated individuals, every year someone spends in prison, it is estimated that their life expectancy is reduced by two years. Despite their scale and impact, prisons are among the least transparent and most understudied public institutions in the nation. In response to these issues, the Urban Institute, with support from Arnold Ventures, launched the Prison Research and Innovation Network (PRIN). PRIN is a consortium of five states, each working to establish a model of transparency, accountability, and innovation in a pilot prison. The purpose of PRIN is to use research and data to shine a much-needed light on prison conditions and develop strategies for effective solutions to promote the health and well-being of people who are confined and work behind bars. Central to the PRIN research effort is the commitment to the use of community-engaged research methods. This talk will share the findings, lessons learned, and future directions from Vermont’s PRIN community-engaged research effort to study and innovate in a pilot prison.
April 15th
3:45 PM ET
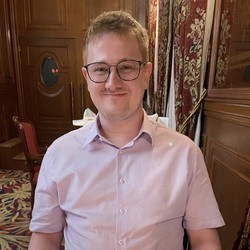
Alec Kirkley
University of Hong Kong
Title: Principled Nonparametric Inference for Network Data Using the Minimum Description Length Principle
Abstract: Networks pose novel challenges for inference and learning due to their discrete, high-dimensional nature. This inherent complexity necessitates the development of statistically principled unsupervised learning objectives that steer clear of ad hoc heuristics to distinguish meaningful structure from noise in real networks. In this talk I will discuss a few recent projects aimed at developing principled unsupervised learning methods that parsimoniously summarize structural and dynamical regularities in network data of multiple forms: geographical networks, multilayer networks, temporal networks, and hypergraphs. These methods are unified under the Minimum Description Length principle from information theory, which readily permits fully nonparametric inference while explicitly highlighting particular regularities of interest in discrete datasets. I will discuss the motivation for this family of methods as well as a general procedure for applying this framework to other problems in network inference. I will also discuss its relationship with hierarchical Bayesian modeling, which allows for the comparison of parameter recovery performance across different optimization algorithms as well as further model selection with posterior predictive checking.
2022-2023 academic year
Fall
September 26th
12:00 PM ET
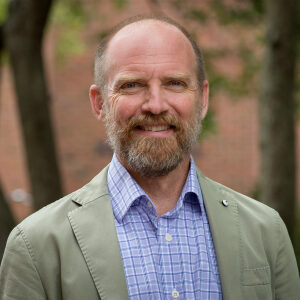
Jeffrey S. Buzas
The University of Vermont
Title: Relations between margin-based binary classifiers and logistic regression
Abstract: This talk explores new connections between logistic regression and margin-based binary classification methods. The connections provide novel perspectives and insight on classification methods that use exponential loss, logistic loss, and other commonly used loss functions. The connections suggest new approaches to adjusting for covariate measurement error in logistic regression with lasso or ridge constraints. Additionally, a general class of loss functions is defined with a population minimizer interpretable on the logit scale. The class includes exponential, logistic, logistic regression, Savage, and α-tunable loss functions, thereby providing additional insight as to their commonalities and differences. An interesting new loss function emerges from the general class. Properties of this new loss function are explored.
October 17th (online)
12:00 PM ET
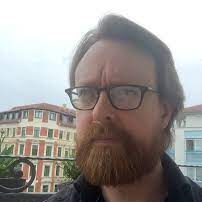
Richard McElreath
Max Planck Institute for Evolutionary Anthropology
Title: Science as Amateur Software Development
Note: We will play a recorded talk, and the speaker will join us for a Q&A session.
November 14th
12:00 PM ET
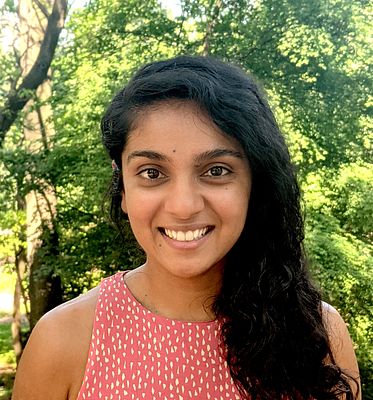
Dhanya Sridhar
Université de Montréal & MILA
Title: Causal inference and machine learning
Abstract: Inferring the effects of changes to a system—causal inference—underpins science and decision-making. Classical techniques for causal inference have relied on carefully measured variables, leaving questions unanswered when measurements are not possible. In contrast, instead of operating on carefully measured inputs, modern machine learning (ML) methods routinely take unstructured data such as text as input and extract task-relevant latent patterns. As such, there is an opportunity for ML to help causality: we can use ML to learn causally-relevant variables from data and use them to study various causal questions. In this talk, I’ll highlight some of our recent work that leverages ML tools like BERT and probabilistic models to draw causal inferences from rich data like text and social networks. I’ll outline the assumptions we’ve developed so far in these settings about when ML can be used to make valid causal inferences. Building on the idea that ML and causality can benefit one another, I’ll also discuss our ongoing work along two threads: 1) a general technical framework on using ML for valid causal inference and 2) how formalisms from causality can help ML generalize better.
Spring
January 30 (online)
12:00 PM ET
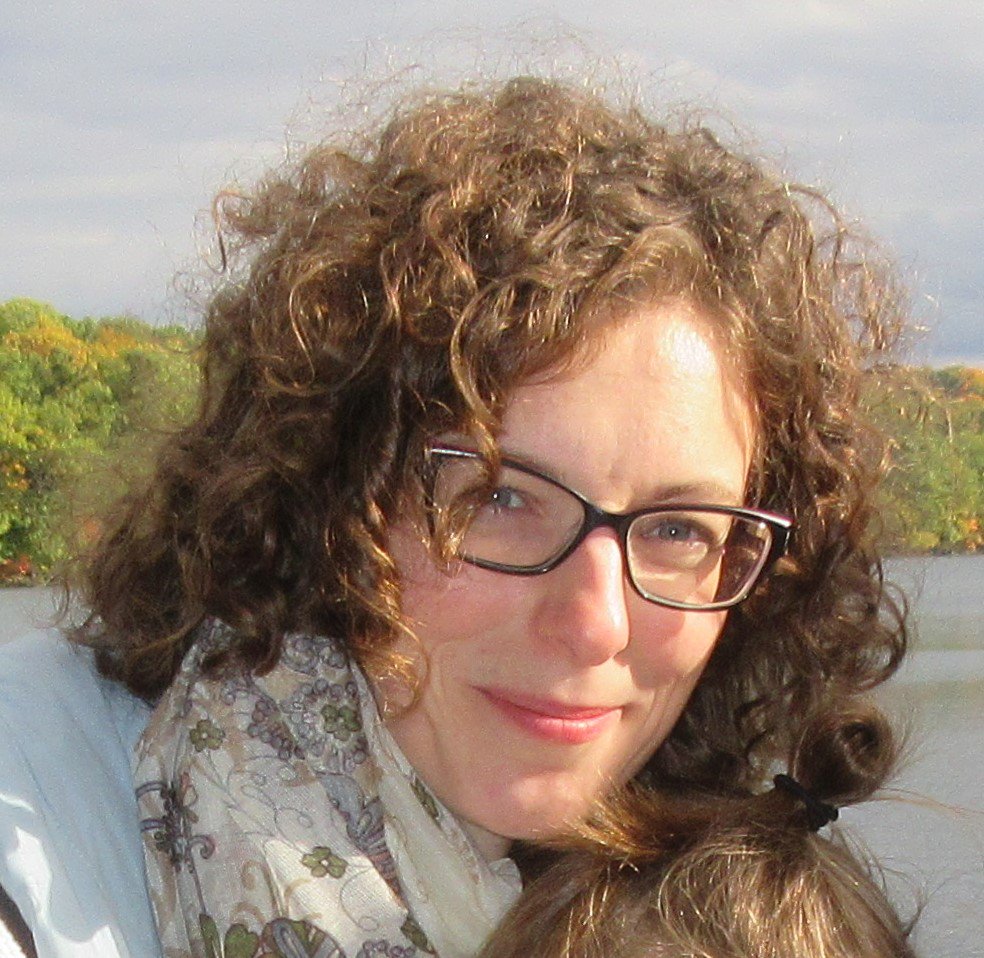
Erica Moodie
McGill University
Title: Penalized doubly-robust estimation of adaptive treatment strategies
Abstract: Adaptive treatment strategies (ATSs) are often estimated from data sources with many covariates measured, only a subset of which are useful for tailoring treatment or control of confounding. In such cases, including all the covariates in the analytic model could possibly yield an inappropriate or needlessly complicated treatment decision. Hence, it is crucial to apply variable selection techniques to ATSs. Variable selection with the objective of optimizing treatment decisions has been the subject of only very little literature. In this talk, I will present a regression-based estimation method that can naturally incorporate variable selection through a penalization approach that incorporates sparsity while ensuring strong heredity and show how we can incorporate confounder selection into the approach. We illustrate the methods using data from a pilot sequential multiple assignment randomized trial of a web-based stress management intervention using a stepped-care method for cardiovascular disease patients to determine useful tailoring variables while adjusting for chance imbalances in important covariates due to the smaller sample size in the pilot. (Joint work with Zeyu Bian, Sahir Bhatnagar, and Susan Shortreed)
February 28th (online)
1:00 PM ET
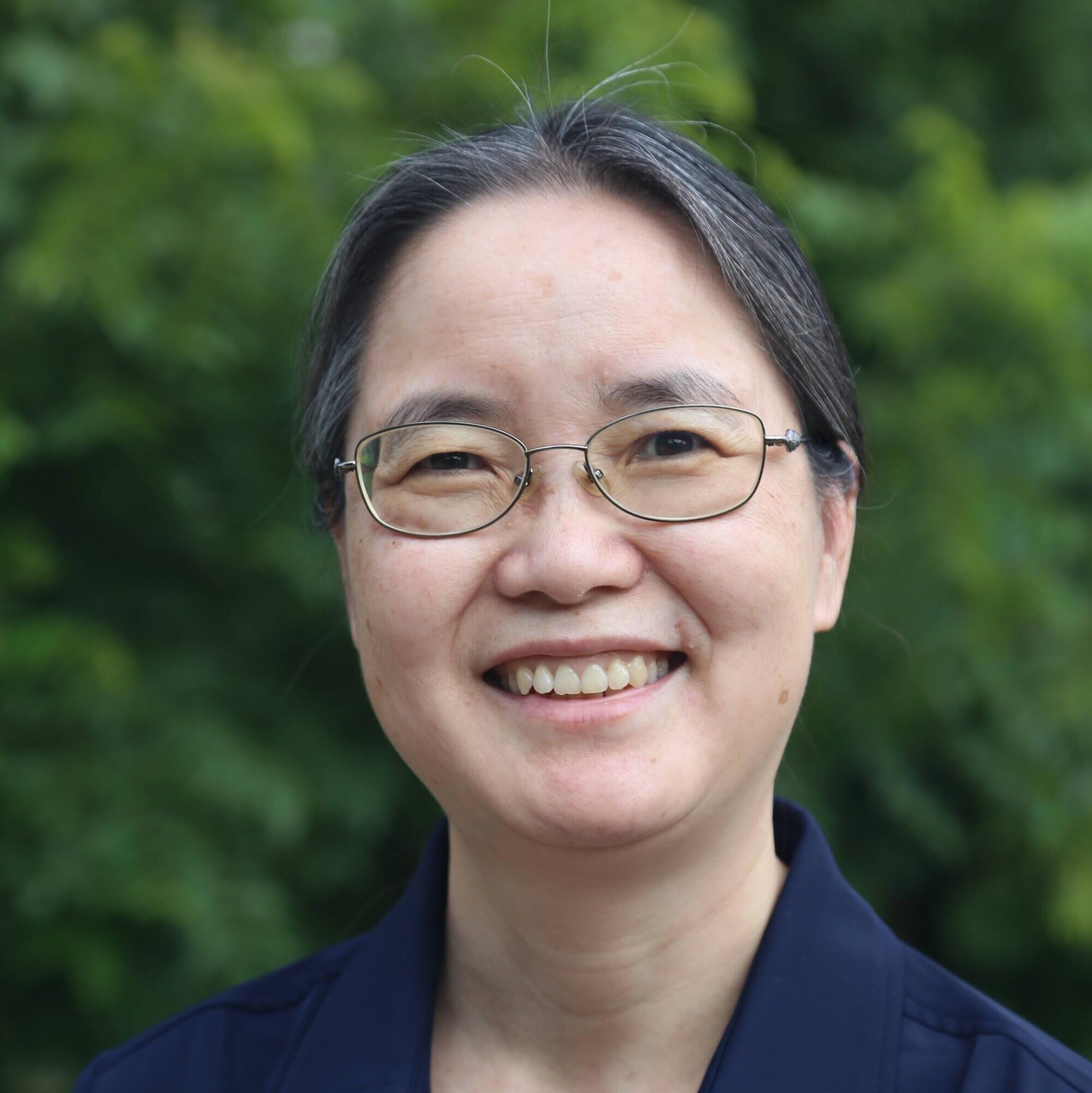
Grace Yi
University of Western University
Title: Boosting Learning of Censored Survival Data
Abstract: Survival data frequently arise from cancer research, biomedical studies, and clinical trials. Survival analysis has attracted extensive research interests in the past five decades. Numerous modeling strategies and inferential procedures have been developed in the literature. In this talk, I will start with a brief introductory overview of classical survival analysis which centers around statistical inference, and then discuss a boosting method which focuses on prediction. While boosting methods have been well known in the field of machine learning, they have also been broadly discussed in the statistical community for various settings, especially for cases with complete data. This talk concerns survival data which typically involve censored responses. Three adjusted loss functions are proposed to address the effects due to right-censored responses where no specific model is imposed, and an unbiased boosting estimation method is developed. Theoretical results, including consistency and convergence, are established. Numerical studies demonstrate the promising finite sample performance of the proposed method.
May 1st
12:00 PM ET
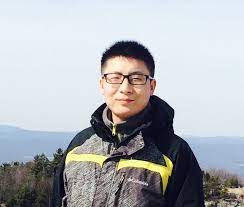
Sen Pei
Columbia University
Title: Bayesian Inference in networked systems and applications in infectious disease modeling
Abstract: Network models are widely used in infectious disease modeling. Many real-world problems need to calibrate high-dimensional network models to observational data. By coupling dynamical network models with real-world data, model-inference systems can support real-time disease forecasting, epidemiological parameter inference, and estimation of unobserved variables. I will introduce several Bayesian inference methods for metapopulation and agent-based models through applications for influenza, COVID-19, and antimicrobial-resistant pathogens.