2022-2023 academic year
Fall
September 26th
12:00 PM ET
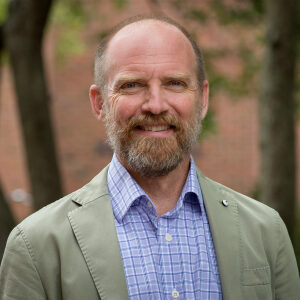
Jeffrey S. Buzas
The University of Vermont
Title: Relations between margin-based binary classifiers and logistic regression
Abstract: This talk explores new connections between logistic regression and margin-based binary classification methods. The connections provide novel perspectives and insight on classification methods that use exponential loss, logistic loss, and other commonly used loss functions. The connections suggest new approaches to adjusting for covariate measurement error in logistic regression with lasso or ridge constraints. Additionally, a general class of loss functions is defined with a population minimizer interpretable on the logit scale. The class includes exponential, logistic, logistic regression, Savage, and α-tunable loss functions, thereby providing additional insight as to their commonalities and differences. An interesting new loss function emerges from the general class. Properties of this new loss function are explored.
October 17th (online)
12:00 PM ET
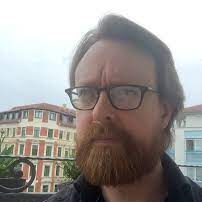
Richard McElreath
Max Planck Institute for Evolutionary Anthropology
Title: Science as Amateur Software Development
Note: We will play a recorded talk, and the speaker will join us for a Q&A session.
November 14th
12:00 PM ET
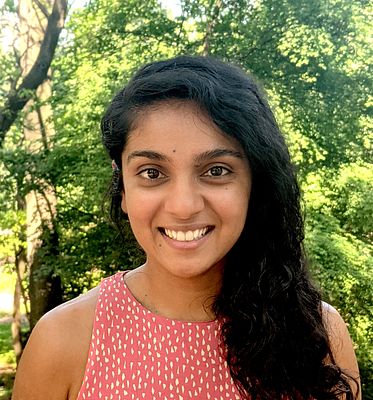
Dhanya Sridhar
Université de Montréal & MILA
Title: Causal inference and machine learning
Abstract: Inferring the effects of changes to a system—causal inference—underpins science and decision-making. Classical techniques for causal inference have relied on carefully measured variables, leaving questions unanswered when measurements are not possible. In contrast, instead of operating on carefully measured inputs, modern machine learning (ML) methods routinely take unstructured data such as text as input and extract task-relevant latent patterns. As such, there is an opportunity for ML to help causality: we can use ML to learn causally-relevant variables from data and use them to study various causal questions. In this talk, I’ll highlight some of our recent work that leverages ML tools like BERT and probabilistic models to draw causal inferences from rich data like text and social networks. I’ll outline the assumptions we’ve developed so far in these settings about when ML can be used to make valid causal inferences. Building on the idea that ML and causality can benefit one another, I’ll also discuss our ongoing work along two threads: 1) a general technical framework on using ML for valid causal inference and 2) how formalisms from causality can help ML generalize better.
Spring
January 30 (online)
12:00 PM ET
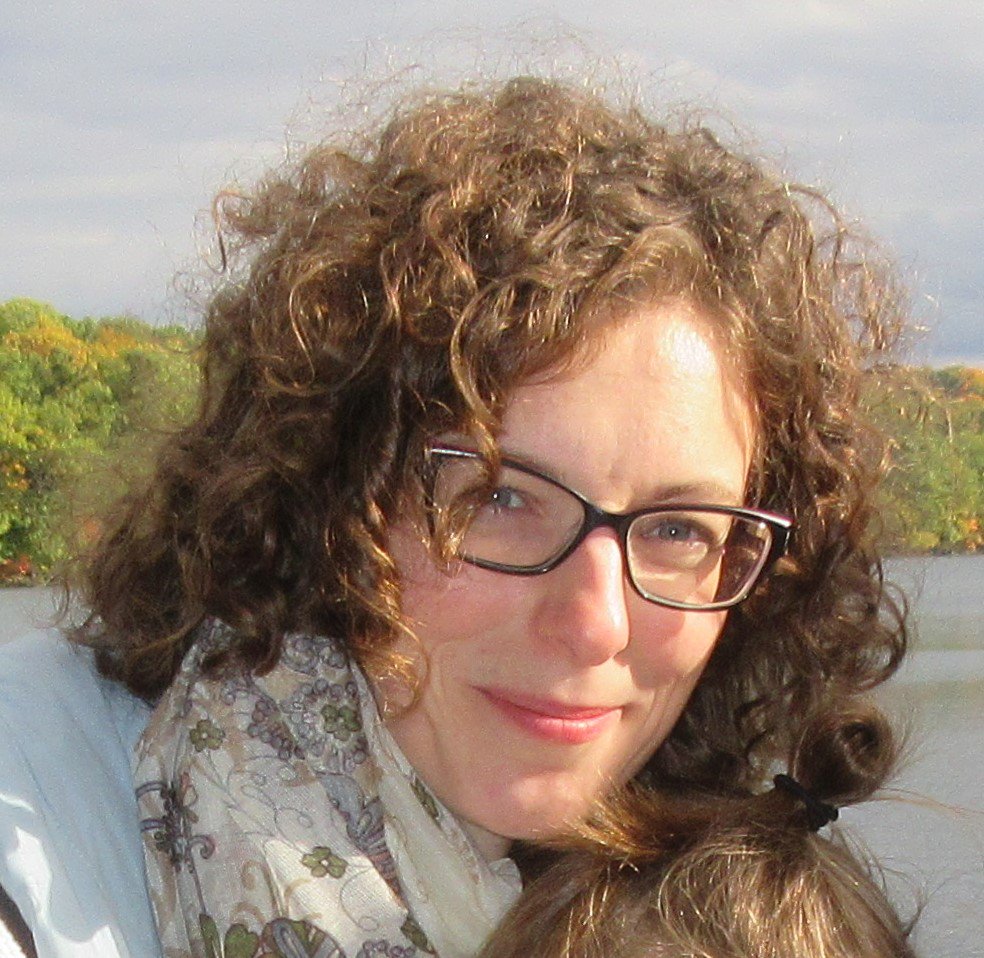
Erica Moodie
McGill University
Title: Penalized doubly-robust estimation of adaptive treatment strategies
Abstract: Adaptive treatment strategies (ATSs) are often estimated from data sources with many covariates measured, only a subset of which are useful for tailoring treatment or control of confounding. In such cases, including all the covariates in the analytic model could possibly yield an inappropriate or needlessly complicated treatment decision. Hence, it is crucial to apply variable selection techniques to ATSs. Variable selection with the objective of optimizing treatment decisions has been the subject of only very little literature. In this talk, I will present a regression-based estimation method that can naturally incorporate variable selection through a penalization approach that incorporates sparsity while ensuring strong heredity and show how we can incorporate confounder selection into the approach. We illustrate the methods using data from a pilot sequential multiple assignment randomized trial of a web-based stress management intervention using a stepped-care method for cardiovascular disease patients to determine useful tailoring variables while adjusting for chance imbalances in important covariates due to the smaller sample size in the pilot. (Joint work with Zeyu Bian, Sahir Bhatnagar, and Susan Shortreed)
February 28th (online)
1:00 PM ET
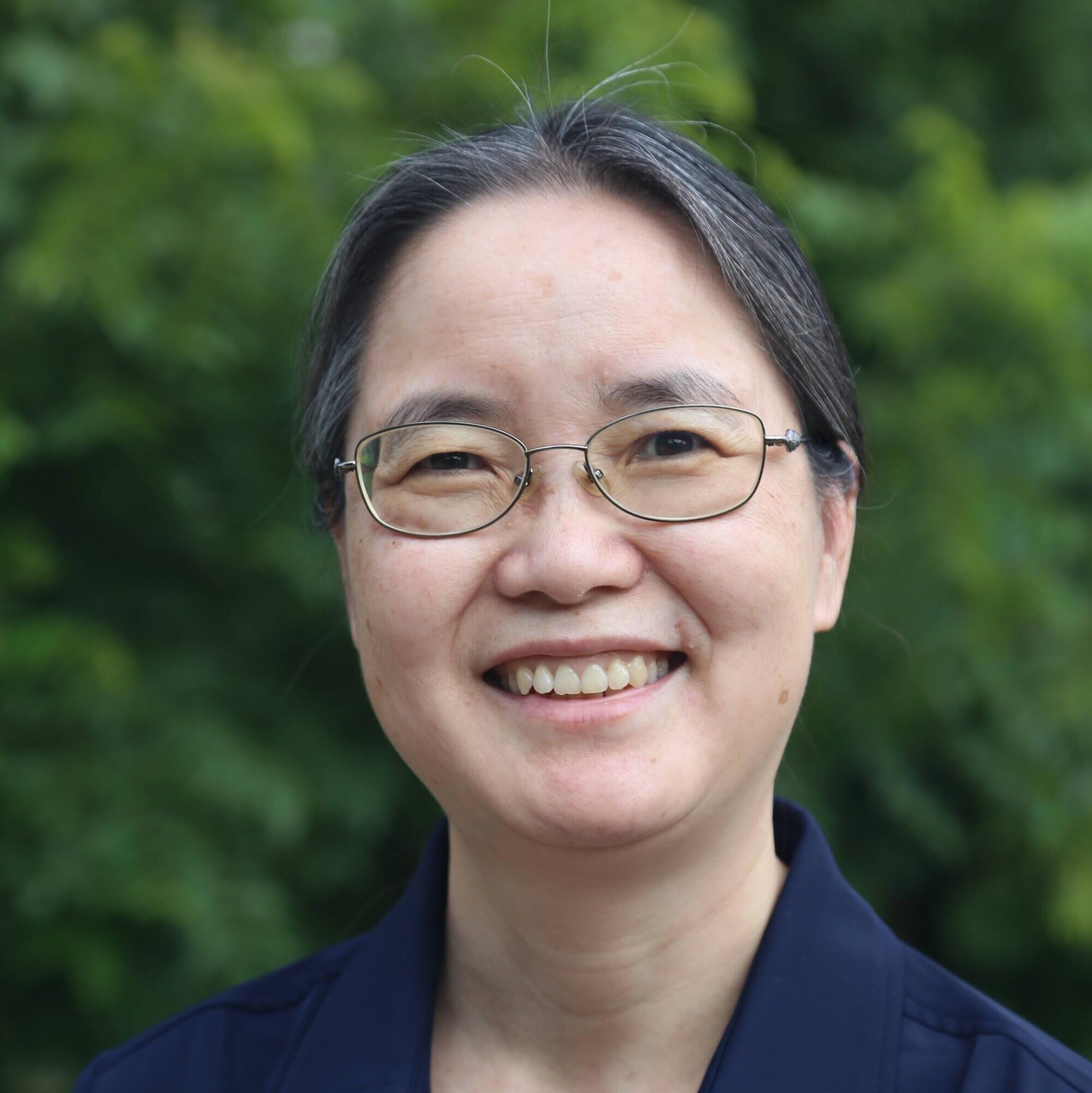
Grace Yi
University of Western University
Title: Boosting Learning of Censored Survival Data
Abstract: Survival data frequently arise from cancer research, biomedical studies, and clinical trials. Survival analysis has attracted extensive research interests in the past five decades. Numerous modeling strategies and inferential procedures have been developed in the literature. In this talk, I will start with a brief introductory overview of classical survival analysis which centers around statistical inference, and then discuss a boosting method which focuses on prediction. While boosting methods have been well known in the field of machine learning, they have also been broadly discussed in the statistical community for various settings, especially for cases with complete data. This talk concerns survival data which typically involve censored responses. Three adjusted loss functions are proposed to address the effects due to right-censored responses where no specific model is imposed, and an unbiased boosting estimation method is developed. Theoretical results, including consistency and convergence, are established. Numerical studies demonstrate the promising finite sample performance of the proposed method.
May 1st
12:00 PM ET
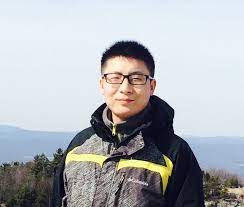
Sen Pei
Columbia University
Title: Bayesian Inference in networked systems and applications in infectious disease modeling
Abstract: Network models are widely used in infectious disease modeling. Many real-world problems need to calibrate high-dimensional network models to observational data. By coupling dynamical network models with real-world data, model-inference systems can support real-time disease forecasting, epidemiological parameter inference, and estimation of unobserved variables. I will introduce several Bayesian inference methods for metapopulation and agent-based models through applications for influenza, COVID-19, and antimicrobial-resistant pathogens.